Published on 2024/01/24 Research powered by Mightex’s Polygon1000
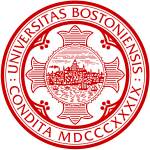
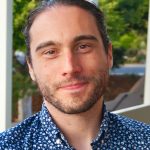
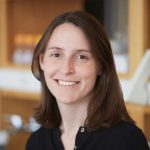
We used the Mightex Polygon digital micromirror device to control gene expression in real time in thousands of single cells via optogenetics. The throughput of the Polygon and its hardware triggering capabilities allowed us to apply hundreds of computer-generated optogenetic stimulation patterns in rapid succession onto our cells. This study was recently accepted in Nature Communications and a preprint is available on bioRxiv: https://www.biorxiv.org/content/10.1101/2022.10.28.514305v1.
We used an Escherichia coli strain that features the CcaSR optogenetic system, which is activated by green light and repressed by red light, to drive a green fluorescent protein’s expression. We grew these cells in a microfluidic device that allowed us to observe the cells over periods of time of over 16 hours using automated time-lapse microscopy (Fig. 1). Every five minutes fluorescence was imaged and quantified for each single cell, and we used an AI-based control algorithm to dynamically decide whether each cell should be exposed to red or green light to drive its fluorescence, and therefore gene expression, towards user-defined objectives (Movie 1).
Figure 1: Schematic of our experimental platform. Cells growing in a microfluidic device are periodically imaged, and a deep model predictive controller decides whether to shine red or green light on each cell.
This AI-based control algorithm is drastically faster at computing the optogenetic stimulation strategies than previous state-of-the art control methods, and the throughput permitted by the Mightex Polygon system allowed us to control thousands of single cells simultaneously, an improvement of over two orders of magnitude over previous approaches, and with better control accuracy.
Figure 2: We controlled 10,000 single cells in real time and generated a dynamic travelling wave pattern.
We demonstrated the capabilities of this platform by controlling 10,000 single cells in real time to reproduce movies, where each pixel in the movie was represented by the fluorescence level
Figure 1 – Schematic of our experimental platform. Cells growing in a microfluidic device are periodically imaged, and a deep model predictive controller decides whether to shine red or green light on each cell.
of a single cell. We first reproduced a 100×100 pixel movie of traveling sinewaves reminiscent of patterns observed in morphogen gradients or communications within biofilms (Fig. 2, Movie 2).
To demonstrate the broad range of temporal patterns that can be applied to each cell, we also reproduced a short clip from the sci-fi classic 2001: A Space Odyssey (Fig. 3, Movie 3). Finally, we applied our approach to a new strain, where the antibiotic resistance gene tetA was co-expressed with the fluorescent protein under the control of the CcaSR optogenetic system. This allowed us to drive resistance levels in single cells in real time and observe and quantify the impact of expression dynamics on cell survival. This insight would not have been accessible without single-cell control of gene expression at high throughput, a feat that was made possible in part thanks to the capabilities of the Mightex Polygon.
To reach this level of throughput, we leveraged two features of the Polygon: First, the ability to load a sequence of several hundreds of images to project into our microscopy field of view. This allowed us to decide whether to illuminate single E. coli cells with red or green light across 80-120 fields of view, and then dynamically generate the sequence of images to load into the Polygon’s memory to illuminate specific cells. We would then sweep through the different positions on our microfluidic chips corresponding to these fields of view, set the Polygon to the appropriate frame in the loaded sequence, and use an external light source (X-Cite XLED1) to illuminate the cells in the field of view with the appropriate red or green light. The second feature of the Polygon that we leveraged was its external triggering and synchronization capabilities. We connected the Polygon to the other devices on our microscope through an Arduino micro-prototyping board. This made it possible to very rapidly trigger and synchronize devices. For each position on our microfluidic device we very quickly switched to the appropriate frame loaded into the Polygon and triggered the relevant red or green LED in the external light source, before moving to the next position. By loading all images at once, and by using external triggering and synchronization, we were able to very rapidly sweep through over 100 positions and apply the appropriate optogenetic stimulation to thousands of single cells every five minutes.
Our research group has been extremely satisfied with not only the quality of the products offered by Mightex, which has been working flawlessly in our hands for 4 years now and offers many important features for our applications, but also of the support provided by the company. Because of the dynamic and computationally demanding nature of our approach, we were delighted to find that not only is the Mightex Polygon 400 supported by the open source microscopy control software Micro-manager, allowing us to implement our entire interactive experimental pipeline in Python, but also that the Mightex support team was willing to help us with our implementation. We have since acquired the more recent Polygon 1000 DMD that we placed on a second microscope to expand our capability of controlling gene expression in real time via AI-based control and optogenetics, and this new model performs even better in terms of resolution, field of illumination, and speed. We are excited to continue working with Mightex products and the Mightex team, and look forward to future developments in their DMD product line and beyond.
Figure 3: We also reproduced a scene from the movie 2001: A Space Odyssey.
Movie 1
Movie 2
Movie 3
Author: Jean-Baptiste Lugagne, PhD
Bio: Jean-Baptiste Lugagne began his academic career in signal processing engineering at Grenoble INP, which led to a transformative encounter with synthetic biology during the iGEM competition in 2011. This experience inspired him to shift his focus, culminating in a Master’s degree at Imperial College where he studied noise in metabolic feedback loops under the guidance of Guy-Bart Stan and Diego Oyarzún. He then embarked on a PhD journey at Université Sorbonne Paris Cité, under the mentorship of Pascal Hersen and Grégory Batt, concentrating on the real-time control of genetic toggle switches.
After earning his PhD, Jean-Baptiste joined Mary Dunlop’s laboratory at Boston University as a postdoctoral researcher in 2018. Here, his work spans a variety of projects, from controlling gene expression to advancing techniques in label-free Raman imaging, image analysis, and studying antibiotic resistance. His research is characterized by a broad interest in quantitative methods for understanding and manipulating cellular processes, leveraging techniques from control theory, machine learning, optics, and synthetic biology. In recent years, Jean-Baptiste has been particularly drawn to the potential of deep learning, especially deep reinforcement learning, for dissecting complex patterns in biological data. He is currently engaged in developing interfaces that integrate cells with advanced AI algorithms, exploring the unique challenges and opportunities at the intersection of biology and artificial intelligence.